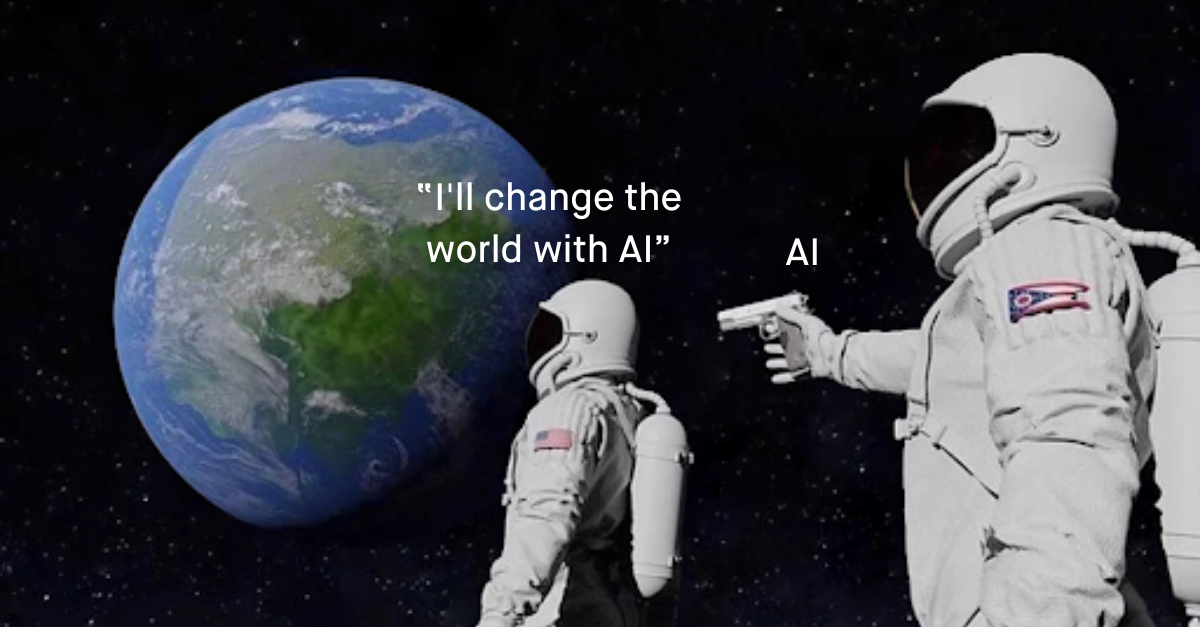
It is no longer a secret…The AI platform shift is here. For builders and investors that have been around long enough to remember the mobile platform shift, companies building products became irrelevant overnight as they became features of a larger platform. We are witnessing a similar phenomenon in the AI platform shift. GPT-4o’s unveiling struck companies building code documentation products, learning products, and much more. This rate of advancement is challenging even the best builders with questions about how to build durable products and valuable companies.
Recently, the founders in our residency program building in/with AI gathered for lunch to unpack these challenges, explore common themes, and probe for answers in terms of how things might shake out and where to find defensibility from competitors and the very pace and nature of AI-innovation itself. See below some specific questions we heard discussed:
Q: How should startups build their tech stack with the sophistication of LLMs that are consistently changing?
A: Within vertical SaaS, the evolution of the modern tech stack is not only about when to use LLMs but also how to use them, which ones to use, and to what end. There is a clear opportunity cost trade-off between spending the team’s time and resources building complicated processes to make today’s elementary LLMs do more advanced tasks compared to spending time building functionality around LLMs that will evolve as model functionality improves. The founders in Grand Central Tech believe the answer here is end-user dependent. Software for mission-critical functions like legal contract review & financial analysis needs increased model complexity to ensure accuracy/reliability, speed, and reduce cost, whereas software for consumer applications is much more straightforward and, therefore, better suited to the LLM creators themselves (see Anthropic with Claude, OpenAI with Chat, etc.). The question for GCT founders remains, “How advanced will foundational models have to disintermediate their existing tech stack?”
Q: What is the new moat in the age of AI, and how are companies going to be able to accrue value over time as the cost of software decreases?
A: Historically, moats have been understood to derive from things like proprietary technology, network effects, economies of scale, patents, and occasionally brand. LLMs within vertical software will likely not be proprietary technology but instead another tool in the tech stack that can automate multi-stage workflows and derive unique insights. Our founders believe, as do we at Company Ventures, that proprietary data combined with LLMs can yield differentiated, sticky products and solutions. At scale, these can unlock network effects and power net new insights.
Q: We’re all vertical SaaS companies. We build workflows to some degree. There's a lot of focus right now on agents and what comes after workflows - there's a gap between the absolute robustness of a workflow and the brittleness of what we're seeing with agents today. What are everyone’s thoughts on the pathway to make agents robust and reliable enough for enterprise use cases?
A: The pathway for AI to move past workflow automation to an agentic format brought up two key roadblocks: 1) Better natural language understanding of code and 2) Improving an agent's ability to understand what a successful workflow looks like. First, as code documentation becomes more prevalent and AI ingests code that is tagged better, the abstractions necessary to instruct an AI agent to work independently will become more prevalent. Second, developing a framework/observability lawyer for AI agents to understand what constitutes a successful execution of a workflow will be critical. With a library of workflows an agent could pick from, these agents could fill in the blanks for customized use cases requiring much less model sophistication and powering reliability for enterprise customers.
Q: How are you framing pricing and value compared to traditional software? How do we think value capture changes in the short and long term?
A: There is already clear evidence of the value that AI-enabled software can deliver to its customers, and the founders in GCT are hyper-focused on capturing more of it. Many founders are building a usage/value-based pricing component into their software pricing to capture value and ensure margin. The ability to increase this value capture is seemingly only possible through the deep customer relationships that helped teams build robust and valuable products in the first place. These relationships are how teams have discovered real pain points for customers, which have proven vital in creating differentiation (especially when larger AI companies are making their products extremely cheap and accessible). How enterprise customer service / the customer-SaaS relationship evolves in the wake of AI may be among the more profound developments we observe.
–
As a firm, we pride ourselves on assembling and executing on the promise of an exceptional venture community. This involves exceptional founders, remarkable space, and thoughtful programming yielding value on a daily basis.
Stay tuned for more insights from our community of technical experts and founders journeying through the fast-changing AI landscape in real-time.
Want to become part of the community? Submit an application for the next Grand Central Tech intake: https://bit.ly/4bxfOm5
Applications close July 8th.